안녕하세요. 이번 리뷰는 조금 독특한 논문을 가져와봤습니다. ERC에 sentiment task도 같이 결합하여 해결하는 방법론인데요. 방법론이 조금 특이해서 읽는데 어려웠던 기억이 있습니다. 그럼 리뷰 시작하도록 하겠습니다.
<1. Introduction>
multimodal machine learning의 급격한 발전과 함께 Multimodal Sentiment Analysis (MSA)와 Emotion Recognition in Conversation (ERC)는 사람의 행동과 intent를 알아차리조 인지하고 이해하는데에 key가 되었다고 합니다. Multimodal data는 verbal information만 제공하는게 아니라 non-verbal information도 제공하는데요. 이러한 다른 modality를 통해 machine은 다양한 관점에서 의사 결정을 내릴 수 있으므로 보다 정확하게 예측할 수 있게 됩니다.
MSA의 목표는 sentiment intensity 또는 polarity를 예측하는 것이고, ERC는 이미 정의된 감정 category를 예측하는 것을 목표로 합니다. MSA와 ERC에 대한 많은 연구 방향들이 있는데 본 논문에서는 대부분의 기존 연구에서 sentiment와 emotion의 유사성과 상호보완성을 무시한 채 MSA와 ERC를 별개의 task로 취급하고 있음을 언급합니다.

한편으로 심리학적인 관점에서 볼 때, sentiment와 emotion은 모두 언어적, 인지적, 사회적 요소의 복합적인 영향에서 비롯되는 경험이며, 유사하게 표현될 수 있습니다. 그림 1에서는 sentiment와 emotion이 언어적 또는 비언어적으로 어떻게 관련되어 있으며, unified embedding space에 투영될 수 있는지를 보여줍니다. 다른 한편으로, 감정은 단기간 내에 현재의 지각된 변화는 반영하는 반면, sentiment는 장기간에 걸쳐 유지돠고 형성된다고 논문에서는 말합니다. 또한 본 논문에서는 사전 연구에서 MSA의 동영상 길이가 ERC의 거의 두 배에 달하는 것으로 나타났음을 말하는데 이는 위의 내용과 일치하는 결과로 받아들일 수 있습니다. 또한 다양한 심리학 문헌에서 sentiment와 emotion 간의 유사성과 차이점을 설명하는데요. 이러한 연구들을 통해서 알 수 있는 점은 sentiment와 emotion을 함께 분석하면 인간의 행동을 더 잘 이해할 수 있다고 합니다.
본 논문에서는 이러한 것에 motivation을 받아, Unified MSA and ERC (UniMSE)라는 multimodal sentiment knowledge-sharing framework에 대해서 제안합니다. UniMSE는 MSA와 ERC를 input, output, task를 통합하는 generative task로 재구성하여 수행합니다. audio와 video feature를 추출하고 통합하여 MSA와 ERC label을 Universal Label (UL)로 공식화하여 emotion과 sentiment를 통합합니다.
게다가 본 논문에서는 pre-trained modality fusion layer (PMF)를 제안하고, 이를 T5의 Transformer layer에 포함시며 보다 풍부한 정보를 탐색하기 위해 acoustic, visual information를 다른 level textual feature와 융합합니다. 마지막으로 inter-modal contrastive learning(CL)을 수행하여 inter-class variance를 최소화하고 inter-class variance를 최대화하도록 합니다.
contribution을 정리하면 아래와 같습니다.
- We propose a multimodal sentiment- knowledge sharing framework2 (UniMSE) that unifies MSA and ERC tasks. The proposed method exploits the similarities and complementaries between sentiments and emotions for better prediction.
- We fuse multimodal representation from multi-level textual information by injecting acoustic and visual signals into the T5 model. Meanwhile, we utilize inter-modality contrastive learning to obtain discriminative multimodal representations.
- Experimental results demonstrate that UniMSE achieves a new state-of-the-art performance on four public benchmark datasets, MOSI, MOSEI, MELD and IEMOCAP, for MSA and ERC tasks.
- To the best of our knowledge, we are the first to solve MSA and ERC in a generative fashion, and the first to use unified audio and video features across MSA and ERC tasks.
<2. Related Work>
<Multimodal Sentiment Analysis (MSA) >
MSA는 multimodal 셋팅에서 sentiment polarity와 sentiment intensity를 예측하는 것을 목표로 합니다. MSA 연구는 4개의 그룹으로 나눠 설명드릴 수 있는데요. 첫번째 그룹은 multimodal fusion입니다. multimodal fusion의 초기 연구들은 대부분 feature spaces에서 geometric manipulation을 수행했습니다. 최근 연구들은 reconstruction loss나 hierarchical mutual information maximixation 기법을 개발하여서 multimodal representation을 최적화합니다. 두 번째 그룹은 multi-task joint learning이나 한 modality에서 다른 modality로 translating을 통해 modal consistency과 difference에 초점을 맞춰 연구합니다. 세 번째 그룹은 multimodal alignment에 초점을 맞췄는데요. cross-modality와 multi-scale modality representation을 활용하여 modal alignment를 구현합니다. 마지막 그룹은 multimodal context에 unimodal context를 통합하는데, 발화 간의 cotext information을 포착하기 위해서 multi-level multiple attentions를 사용하기도 했다고 합니다.
<Emotion Recognition in Conversation (ERC) >
dialogue system에 대한 연구 관심이 증가함에 따라, 대화에서 predefined된 감정을 인식하는 방법이 연구의 hotspot이 되고 있다고 논문에서는 말하는데요. 게다가 multimodal machine learning이 떠오름과 함께, ERC 연구는 멀티모달 패러다임으로 확장 되었다고 합니다. multimodal emotion recognition in conversation은 큰 진전을 하게 되었는데요. 연구 방향은 mulfimodal fusion, context-aware models, external knowledge 통합으로 분류할 수 있습니다. Hu, Joshi 연구자 등은 발화 또는 화자의 inter/intra dependencies를 모델링하기 위해 graph neural network를 채택하였구요. 다른 연구자는 문맥 통합(context incorporation)을 위해서 graph 구조로 구성하여 context를 모델링하였고, 또 다른 연구자는 emotion dynamics 개념을 도입하여서 context를 포착하였습니다. 게다가, 일부 발전된 ERC에서는 외부 지식이 통합하여 사용하였는데요. 여기서 말하는 외부 지식은 transfer learning, commonsense knowledge, multi-task learning, external information을 말합니다.
<Unified Framework>
논문에서는 최근 몇 년동안 관련성이 있지만 서로 다른 작업을 하나의 프레임워크로 통합하는 것이 상당히 발전했다고 말합니다. 예를 들어서, T5라는 언어모델이 있는데요. T5는 모든 텍스트 기반의 language problem을 text-to-text format으로 캐스팅하여 다양한 NLP Task를 통합하고 많은 벤치마크에서 SOTA를 달성했습니다. 더 최근에는, unified index generateive 방식으로 모든 ABSA task를 해결한 것도 있고, unified generative dialogue understanding 프레임워크를 제안한 연구자가 있는 등 unifined 프레임워크를 사용한 연구가 많은 주목을 받고 있다고 합니다. 이러한 것을 통틀어 보면 모델 성능과 일반화를 개선하는 데 있어 이러한 통합 프레임워크의 우수성을 보여주는데요. 본 논문에서는 T5를 백본으로 사용하여 MSA와 ERC를 통합하고 이 프레임워크에서 통합된 임베딩 공간을 학습합니다.
<3. Method>
<3.1 Overall Architecture>

Figure 2를 통해서 UniMSE의 전반적인 모델 구조를 확인할 수 있는데요. UniMSE는 task formalization, pre-trained modality fusion, inter-modality contrastive learning으로 구성되어 있습니다. 먼저, MSA와 ERC task의 label을 off-line에서 universal label(UL) format으로 변환시킵니다. 그런 다음 데이터셋간의 unified feature extractor를 사용하여 오디오와 비디오 feature를 별도로 추출합니다. 오디오, 비디오 feature를 추출한 후에는 두 개의 개별 LSTM에 feed하여 long-term contextual information을 사용할 수 있도록 합니다. text modality의 경우, sequence의 contextual information을 학습하기 위해 T5를 encoder로 사용합니다. 그리고 T5에 multimodal fusion layer를 임베딩하여 T5의 여러 transformer layer 각각에서 feed-forward layer를 통과하도록 합니다. 게다가, sample간 multimodal fusion representation을 구별하기 위해서 inter-modal contrastive learning을 수행합니다. 특히, contrastrive learning은 같은 sample의 modality 간 격차를 좁히고 서로 다른 sample의 modality representation은 더 멀리 떨어뜨리는 것을 목표로 합니다.
<3.2 Task Formalization>
vledeo frame을 i라고 했을 때 mutlimodal signal을 I_i = [I^t_i, I^a_t, I^v_t]라고 할 수 있습니다. 여기서 t, a, v는 text, acoustic, visual이라고 말할 수 있구요. MSA는 sentiment strength를 반영하는 real number y_i^r \in \mathbb{R}을 예측하는 것을 목표로하고, ERC는 각 발화의 emotion category를 예측하는 것을 목포로 합니다. MSA와 ERC는 task formalization을 통해 input feature, model architecture, label space에서 unified됩니다. Task formalization은 input formalization과 label formalization가 있는데요. input formalization은 dialogue text와 modal feature를 처리하는데 사용되고, label formalization은 MSA와 ERC task에 해당하는 레이블을 universal label로 변환시켜 두 task를 통합하는데 사용됩니다. 또한 논문에서는 MSA와 ERC를 single architecture로 합치기 위해서 generative task로 formalize합니다.
<3.2.1 Input Formalization>

대화에서 contextual information은 특히 사람의 감정과 intent를 이해하는데 중요한데요. 이러한 observation을 기반으로, 현재 대화 u_i의 2-turn 이전의 utterance {u_{i-1}, u_{i-2}}와 2-turn 이후의 utterance {u_{i+1}, u_{i+2}}를 raw text로 concat하여 가져갑니다. 여기에, segment id S_i^t를 설정해주는데요. utterance u_i와 u_i의 contexts를 구분하기 위해서 사용합니다. 위의 (1)을 통해서 어떻게 I가 구성되고 S가 구성되는지 확인할 수 있습니다. 오디오의 경우 이렇게 처리하는데요. raw acoustic을 input으로 받으면 librosa라는 음성 처리 라이브러리를 통해서 Mel-spectrogram으로 변환하는데요, 이를 audio feature로 사용합니다. 비디오의 경우, 각 segment에서 고정된 T frame을 추출하고 VGGface 및 AFEW 데이터셋에 대해 사전학습 된 effecientNet을 사용하여서 video feature를 얻습니다.
<3.2.2 Label Formalization>
본 논문에서는 MSA와 ERC 간의 information boundary를 허물기 위해서 universal label (UL)을 디자인하였는데요. 이 UL을 UniMSE의 target sequence로 삼습니다. universal label은 sentiment와 emotion에 대한 MSA와 ERC 간의 shared knowledge를 완전히 탐색하는 것을 목표로합니다.
universal label y_i = {y^p_i, y^r_i, y^c_i}는 이렇게 주어지는 데요. sentiment polarity y_i^p \in {positive, negative, neutral} , sentiment intensity y^r_i, 그리고 emotion category y_i^c로 구성됩니다. 여기서 sentiment intensity는 MSA의 supervision signal로 -3에서 3까지의 범위를 가지는 real number로 구성되어 있으며, emotion category는 ERC의 supervision signal로 미리 정의된 emotion category를 의미합니다.
본 논문의 저자는 annotation을 추가로 달았는데요. 비슷한 semantics(ex: 1.6과 joy)로 sample을 정렬하여 하나는 sentiment intensity로 annotation하고, 다른 하나는 emotion category로 annotation 했다고 합니다. label space를 alignment 한 뒤에, 각 sample의 label은 universal label format으로 formalize됩니다.
다음은 label space에서 MSA와 ERC task를 unify하는 방법을 소개드리고자 합니다.

처음으로, 먼저 sentiment polarity에 따라 MSA와 ERC의 sample을 positive, neutral, negative sample set으로 분류합니다. 그런다음 같은 sentiment polarity이지만 다른 annotation scheme에 속하는 두개의 sample간의 유사도를 계산하여 universal label에서 missing된 part를 완성합니다. 이렇게 말로만 설명 들으면 이해가 잘 가지 않는데요. Figure 3를 통해서 예를 확인할 수 있습니다. MSA sample m_2가 주어진다고 해봅시다. 이 sample은 positive sentiment와 1.6의 annotation score를 가지고 있습니다. m_2에는 emotion category label이 없는데요. 이 예에서는 m_2와 semantic similarity가 가장 높은 e_1를 선택한 다음, e_1의 emotion category를 m_2의 emotion category로 할당합니다.
본 논문에서는 이전 연구에서 이미 text modality가 다른 modality보다 더 많은 정보를 제공한다는 것이 입증되었기 때문에 textual similarity를 sample 간의 semantic similarity로 채택히여 사용하였습니다. 특히, strong sentence embedding framework SimCSE를 활용하여서 universal label을 완성하기 위해서 두 text의 semantic similarity를 계산합니다. 비슷하게 ERC 데이터셋에 있는 sample은 가장 비슷한 MSA sample을 계산함으로서 real number를 할당시킵니다. 이렇게 formalization 후에는, MSA, ERC sample은 {(I_0, y_0), (I_1, y_1) ... (I_N, y_N)}로 바뀝니다. 여기서 I_i는 sample i의 raw multimodal signal을 의미하며 y_i는 universal label을 의미합니다. 예측된 UL을 decoding하여 MSA와 ERC의 예측값(predictions)를 얻을 수 있습니다. 게다가, universal labels에서 자동으로 생성된 부분의 성능을 평가하는데 논문의 저자들이 MOSI 데이터셋에서 universal label이 있는 80개의 sample을 랜덤하게 선정하여 universal label 완성에 사용된 생성된 label을 수동으로 평가를 진행햇었는데요. 정확도는 90%에 달했다고 합니다. 이 말은 잘 universal label을 생성하고 있다라고 받아 들일 수 있는 부분 같습니다.
< 3.3 Pre-trained Modality Fusion (PMF) >
본 논문 이전의 논문들은 T5와 같은 사전학습 모델을 단순히 text encoder로만 사용하였는데, 본 논문에서는 multimodal fusion layer를 사전학습 모델에 임베딩하여 사용하였습니다. 이 때문에 acoustic, visual signal이 text encoding에 참여할 수 있고, multiple level의 textual information과 융합될 수 있습니다. 얕은 Transformer layer로 인코딩된 low-level의 text syntax feature와 깊은 Transformer layer로 인코딩된 high-level의 text semantic feature는 acoustic feature와 video feature와 융합되어 multimodal representation으로 변환됩니다. 게다가, T5에 audio와 vision을 주입하면 방대한 text knowledge에서 관련 정보를 probe할 수 있으므로, 풍부한 pretrained understanding을 multimodal fusion representation에 통합할 수 있습니다. 본 논문에서는 이러한 multimodal fusion process를 pre-trained modality fusion (PMF)로 이름 지었습니다.
본 논문에서는 UniMSE의 백본으로 T5를 사용합니다. 잠시 T5에 대해서 설명 드리자면, T5는 여러개의 stacked Transformer layer가 포함되어 있으며, encoder와 decoder를 위한 Transformer layer는 feedforward layer를 포함하고 있습니다. multimodal fusion layer는 feedforward layer 후에 follow되도록 설정되어 있는데요. 기본적으로 T5의 첫 번째 Transformer layer에 있는 PMF unit은 입력으로 triplet M_i = (X_i^t, X_i^a, X_i^v)를 가집니다. 여기서 X_i^m \in R^{l_m \times{d_m}}은 [/latex]I_i^m , m \in {t, a, v}의 modality representation을 의미하고, [latex]l_m과 d_m은 modality m의 sequence length와 representation dimension을 의미합니다. 본 논문에서는 adapter로 multimodal fusion layer를 간주하고 이를 T5 모델에 삽입하여 multimodal fusion을 위한 specific. parameters를 최족화합니다. multimodal fusion layer는 modal representation triplet M_i를 받고, multimodal concatenation representation의 size를 layer의 input size로 다시 맵핑합니다. 구체적으로 말씀드리자면, 세 가지 modal representation을 concat한 다음 concat한 것을 down-projection layer와 up-projection layer로 feed하여 representation을 fusion 합니다. j번째 PMF에 대해서, multimodal fusion은 아래 (2)와 같이 표현할 수 있습니다.

여기에서 X_i^{a,l_a} \in R^{l\times{d_a}}와 X_i^{v,l_v} \in R^{l\times{d_v}}는 X_i^a와 X_i^v의 마지막 time step의 hidden state를 의미합니다. X_i^a와 X_i^v는 두개의 각각의 LSTM으로 인코딩된 acoustic, visual modality representation을 의미합니다. [\dot]은 feature dim에서 concat operation을 의미하고, σ는 sigmoid를 의미합니다. {W^d, W^u, W, b^d, b^u}는 learnable parameter를 의미합니다.
F_i^{(0)} = X_i^t이고 X_i^t는 T5의 첫번째 Transformer layer로부터 인코딩된 text representation을 의미하는데요. F_i^{(j-1)}은 (j-1) Transformer layer 후에 fusion representation을 의미합니다. ⊙ 은 element addition을 의미합니다. fusion layer의 output은 바로 layer normalization을 통과하게 됩니다.
본 논문에서는 T5의 인코더와 디코더의 각 Transformer에 multmodal fusion layer를 임베딩할 수 있지만 이러한 경우 두가지 단점이 있을 수 있다고 합니다.
- text sequences의 encoding을 방해한다
- multimodal fusion layer에 더 많은 파라미터가 설정되어서 과적합이 발생 할 수 있다
이러한 문제를 본 논문에서는 고려하여 former j Transformer layer를 사용하여 text를 encoding하고 나머지 Transformer layer에는 non-verbal( 예를 들어서 acoustic, visual) signal를 넣습니다.
< 3.4 Inter-modality Contrastive Learning >
Contrastive learning (CL)은 sample을 여러 관점에서 바라봄으로써 representation learning에서 큰 발전을 이루었다고 하는데요. contrastive learning의 원리는 feature space에서 anchor와 positive sample은 더 가까이 끌어당기고, anchor와 negative sample은 더 멀리 밀어내는 것으로 말할 수 있습니다. 본 논문에서는, modality간 contrastive learning을 수행하여 modality 간의 상호작용을 향상시키고 sample간의 fusion representation의 differentiation을 최대화 합니다. 또한, input sequence의 각 element가 해당 context를 인식할 수 있도록, 각 modal representation을 동일한 sequence length로 처리합니다. 그런 다음 1D temporal convolutional layer를 통해 acoustic representation X_i^a, visual representation X_i^v, fusion representation F_i^{(j)}를 전달합니다.

F^{(j)}_i는 j Transformer layer 후에 얻는 값인데요. k^u (u ∈ {a, v})는 modality u에 대한 convolutional kernel의 크기를 의미하고, k^f는 fusion modality에 대한 convolutional kernel의 크기를 나타냅니다.
각 mini-batch는 k개의 sample로 구성됩니다. 물론 여기서 각 sample은 acoustic, visual, text modality로 구성되어 있습니다. 위에서 말씀드린데로 이 논문 이전에 연구에서 이미 text modality가 다른 두 modality에 비해서 더 중요하다는 것이 입증 되었기 때문에 text modality를 anchor로 삼고 다른 두 modality를 agumented version으로 삼습니다.
각 anchor에 대해서 랜덤하게 샘플링된 pair의 batch는 두 개의 posotive pair와 2k negative pari로 구성됩니다. 여기서 positive sample은 동일한 sample에서 text와 acoustic으로 구성된 modality의 pair이고, negative sample은 동일한 sample에서 text와 visual로 구성된 modality의 pair입니다. negative sample의 example로는 text와 다른 sample의 다른 두 가지 modlaity로 구성된 modaliy pair라고 말할 수 있습니다. 각 anchor sample에 대해 self-supervised contrastive loss는 다음과 같이 공식화해서 말할 수 있습니다.
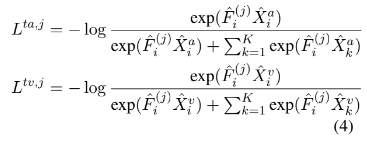
여기서 L^{ta, j}와 L^{tv,j}는 encoder의 j번째 Transformer layer에서 수행되는 text-acoustic, text-visual의 contrastive loss를 나타냅니다.
< 3.5 Grounding UL to MSA and ERC >
training 동안에는 negative log-likelihood를 사용하여 모델을 최적화하는데요. universal lavel을 target sequence로 취해서 최적화합니다. 전체 loss function은 아래와 같습니다.

L^{task}는 generative task loss를 의미하고 j는 Transformer layer of the Encoder의 index를, {α, β}는 0과 1 사이의 소수점으로 weight value를 의미합니다.
< 4. Experiments >
< 4.1 Datasets >
본 논문에서는 멀티모달 감정인식 논문에서 한번 봤다 하는 데이터셋을 모두 사용하였는데요. 아래와 같이 사용하였습니다.
- MOSI (Multimodal Opinion-lvel Sentiment Intensity dataset)
- MOSI는 sentiment score가 -3에서 3까지로 측정되어 있는 데이터셋입니다.
- MOSEI (Multimodal Opinion Sentiment and Emotion Intensity)
- MOSEI는 MOSI의 업그레이드 버전이라고 생각하시면 됩니다. sentiment와 emotion이 동시에 라벨링 되어있습니다.
- MELD (Multimodal EmotionLines Dataset)
- MELD는 multi-party conversation의 video clip으로 구성되어 있는데요. 6감정으로 구성되어있다고 합니다.
- IEMOCAP (Interactive Emotional dyadic Motion CAPture database)
- 본 논문에서는 IEMOCAP 데이터셋에서 6감정만 골라 사용하였다고 합니다.
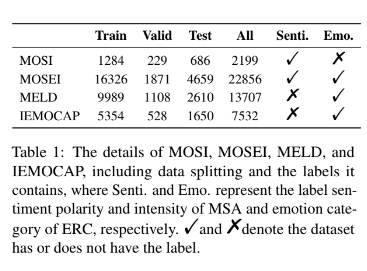
Table 1을 통해서 데이터셋이 어떻게 구성되어 있는지 한눈에 파악할 수 있습니다.
< 4.2 Evaluation metrics >
논문을 보면서 중요한 부분이라면 평가지표가 어떻게 되는지인 것 같습니다. 평가지표는 아래와 같습니다.
- Corr : Pearson correlation
- ACC-7 : seven-class classification accuracy
- ACC-2 : binary classification accuracy
- F1-score : 본 논문에서는 positive/negative와 non-negative/negative classification에 대해서 계산했다고 합니다.
- MELD와 IEMOCAP의 경우, ACC와 weight F1 (WF1)을 사용했습니다.
< 4.3 Results >
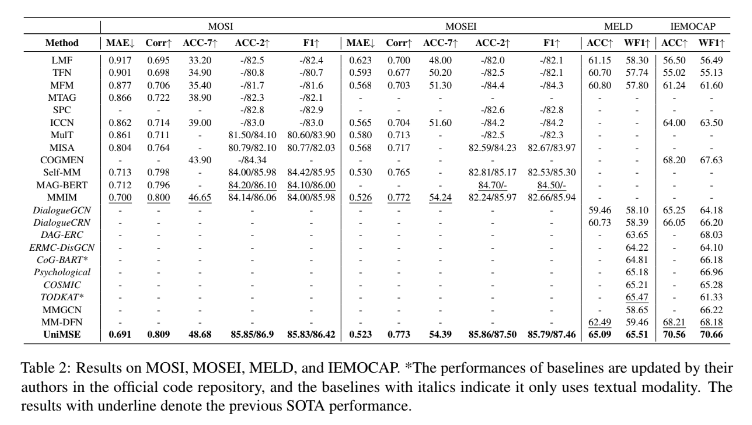
Table 2를 통해서 실험 결과를 확인할 수 있습니다. 처음에 이 테이블을 처음 봤을 때 정말 많은 실험을 진행해서 놀랐는데요. 이 논문이 MSA, ERC 방법론를 모두를 포함하는 방법론을 제안했기 때문에 MSA 방법론들이랑 ERC 방법론들을 모두 실험한 것 같더라구요. 대단하다는 생각밖에 들지 않습니다. 그리고 더 대단한 점은 모든 방법론에서 성능 향상을 이루었다는 것입니다. 본 논문에서는 이러한 성능 향상을 통해 MSA, ERC task에서 UniMSE가 우수하다는 것을 보여주고 dataset과 task간의 knowledge sharing에서 unified framework 효과를 입증한다고 말합니다.
< 4.4 ablation Study >
사실 성능 향상을 이룬 것 만큼이나 더 중요한 부분은 ablation study가 되겠는데요. 본 논문에서 진행한 ablation study는 아래의 표로 확인할 수 있습니다.
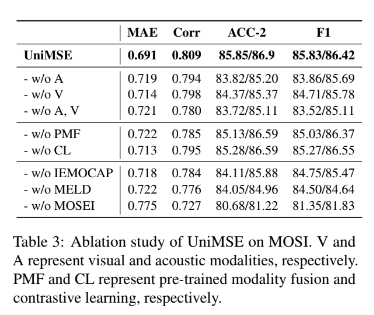
본 논문에서는 MOSI에서 ablation study를 진행했는데요. 우선 먼저 여러개 modality를 사용한 것에서 modality 하나를 제거하거나 하나만 사용한다거나 하는 식으로 실험을 수행하였는데요. 표에서 볼 수 있듯이 visual modality를 제거하거나 acoustic modality를 제거하거나 모두 제거하면 성능이 저하되는 것을 확인할 수 있고, 이를 통해서 비언어적 신호(시각, 청각을 말합니다)가 MSA task를 해결하는 것에 필요하고 text, acoustic, visual modality가 상호 보완적이라는 것을 확인할 수 있습니다.
다음으로 실험을 진행한 것은 UniMSE에서 PMF와 CL 모듈을 제거한 것인데요. 이러한 경우 MAE는 증가하고 Corr은 감소한 것을 확인할 수 있습니다. 이러한 결과는 multimodal representation learning에서 PMF와 CL이 효과적이라는 것을 보여줍니다.
다음으로는 데이터셋이 UniMSE에 미치는 영향을 확인하기 위해서 실험을 진행하였는데요. training dataset에서 IEMOCAP, MELD, MOSEI를 제거하고 MOSI test dataset에서 성능을 확인하였는데요. IEMOCAP와 MELD를 제거하면 MAE와 Corr에서 성능이 저하되는 것을 확인할 수 있는데, 이 결과는 MELD/IEMOCAP을 제거함으로써 MSA 작업에 제공하는 정보가 줄어들어 그런 것이라 논문에서는 추측하였습니다.
이렇게 리뷰를 마치는데요. 이번 논문은 MAE와 ERC를 동시에 해결하기 위해서 Universal label을 만든다는 것이 흥미로웠던 논문입니다. 이렇게 새롭게 데이터셋을 만들어 task를 해결할 수도 있다는 것을 알게 해준 논문 이었던 것 같습니다. 읽어주셔서 감사합니다.
안녕하세요 좋은 리뷰 감사합니다.
서로 다른 두 task인 MSA와 ERC를 하나의 네트워크에서 수행하면서 각 모달리티의 정보를 상호 보완적으로 활용하여 결국 양쪽의 성능이 모두 올라간 것이 인상적이었습니다. 또한 이를 위해 새롭게 labeling을 진행하는 방식을 제안한 것 역시 신기하네요,
간단한 질문이 있는데 제가 잘 아는 것은 아니지만 ERC는 대화 상황에서 utterence단위로 A,V,T데이터가 존재하고 각각에 대해 emotion이 라벨링 되어 있어 해당 감정을 classification으로 맞추는 task로 알고 있습니다. 그렇다면 MSA task에 대해 보중 설명해 주실 수 있을까요? sentiment intensity를 예측한다는 언급만 있어서 잘 와닿지 않는 것 같습니다.
안녕하세요. 댓글 감사합니다.
sentiment analysis와 emotion recognition의 차이점을 말씀 드리는게 더 설명에 좋을 것 같네요. emotion recogntion 같은 경우, 감정의 카테고리 즉, 슬픔, 행복 이러한 감정을 classification하는 것을 말하고, sentiment analysis의 경우, 감정의 격함, 긍정인지 부정인지와 같은 것을 의미합니다.
감사합니다.