요약:
본 논문은 모델의 representation 능력을 강인하게 하여 noise가 포함된 데이터에서 학습을 진행할 수 있도록한다.
Introduction
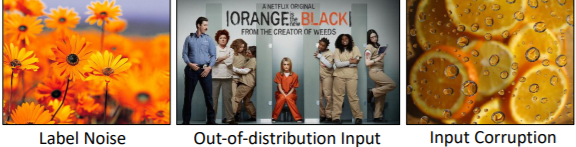
기존의 DNNs은 noise에 민감하였다. 또한 real world data는 주로 noise가 포함된 것이 많으며 [그림1]과 같이 다양한 종류의 noise가 있다. 그 중 기존의 연구는 보통 Input Corruption을 noise로 가정하여 이를 해결하고자 하였다. 본 논문은 세가지 noise에 모두 대응하기 위한 noise-robust contrastive learning과 noise cleaning method를 준비하였다. 먼저 noise-robust contrastive learning은 unsupervised consistency contrastive loss와 weakly supervised prototypical contrastive loss로 구성되었다. 다음으로 noise cleaning은 top-k개의 이웃 예측을 통해 pseudo-label를 생성하는 것이다. 자세한 method에 대한 설명은 다음과 같다.
Method
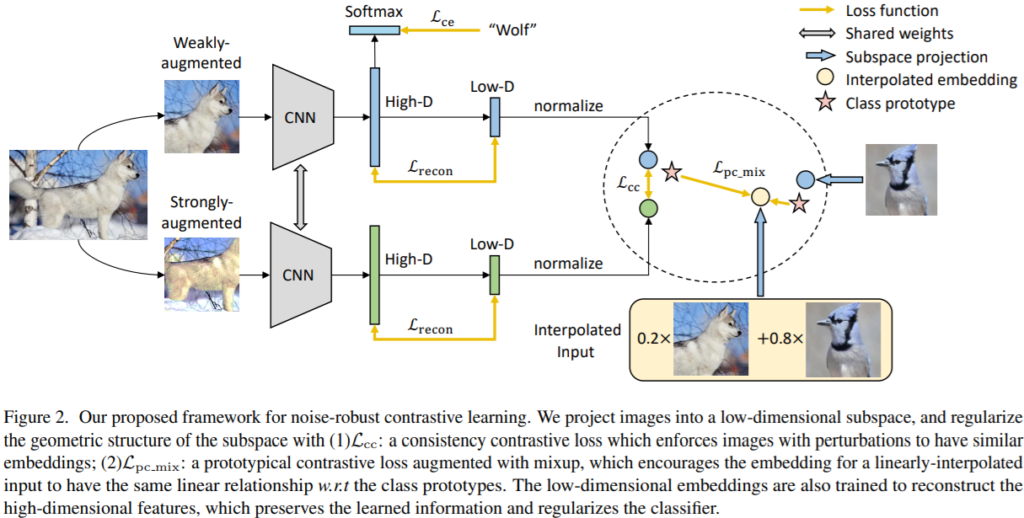
Noise-robust contrastive learning – Unsupervised consistency contrastive loss
목적: 의미론적으로 유사한 이미지에 대해 유사한 embedding을 갖도록 한다.
방법: strong augmentation을 적용한 Z_j와 weakly augmentation(filp, shift)를 적용한 Z_i의 embedding 분포가 유사해지도록 loss를 구성한다.
Noise-robust contrastive learning – Weakly-supervised mixup prototypical contrastive loss
목적: 이미지의 최종 embedding인 Z~_i가 클래스의 prototype(클래스에 해당하는 데이터의 평균으로 구한 사전지식), Z~_c 와 유사해지도록 학습
방법: 먼저 class c에 해당하는 이미지 I_c를 labeled data인 D에서 추출하여 수식1을 통해 class prototype, Z~_C를 구한다. 다음으로 noise를 포함할 후 있는 y_i를 수식2를 통해 정규화할 수 있도록 학습한다. 더욱 확실한 정규화를 위해 해당 연구는 두 개 이상의 학습데이터를 가중치(λ) 결합하여 consistency regularization를 진행하기 위한 학습방식인 Mixup을 수식3과 같이 추가로 적용하며 Weakly-supervised mixup prototypical contrastive loss를 구성하였다.
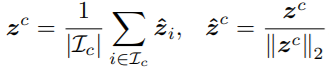


Noise-robust contrastive learning
위의 두가지 외에도 low-dimensional embeding(z space)을 위해 autoencoder를 학습하는 loss(Reconstruction loss)와 전반적인 모델의 학습을 위한 Classification loss를 가중치 1로 결합하여 Total loss로 사용한다.
Noise cleaning with smooth neighbors
목적: 데이터에 포함될 수 있는 noise를 top-k개의 neighbors로 smoothing한다.
방법: soft pseudo-label(q_i)로 training sample을 생성하여 학습한다. 이때 top-k개의 이미지를 mixup에 사용하는데 k=200이다. 첫번째 term은 neighbors’ label간의 smoothness를 위하며 두번째 term은 model의 prediction(p)을 유지하기 위해 사용된다. q는 200개의 prediction을 포함한 soft label이며 p는데이터에 대한 모델의 softmax prediction이다.

실험
정량적 결과 noise가 있는 CIFAR datasets을 위한 기존 SOTA method와 비교하였을 때 제안하는 모델이 SOTA를 거두었다. 이때 노이즈 생성을 위해 임의의 n% 데이터를 랜덤 선정하여 랜덤하게 라벨값을 바꾸는 Sym(Symmetric)방식과 특정 클래스쌍 간에(ex. dog<->cat, deer<->horse) 변화가 있는 Asym(Asymmetric)방식을 설계하였다. 각 noise타입은 표의 2행에 Noise type을 참고할 수 있다.
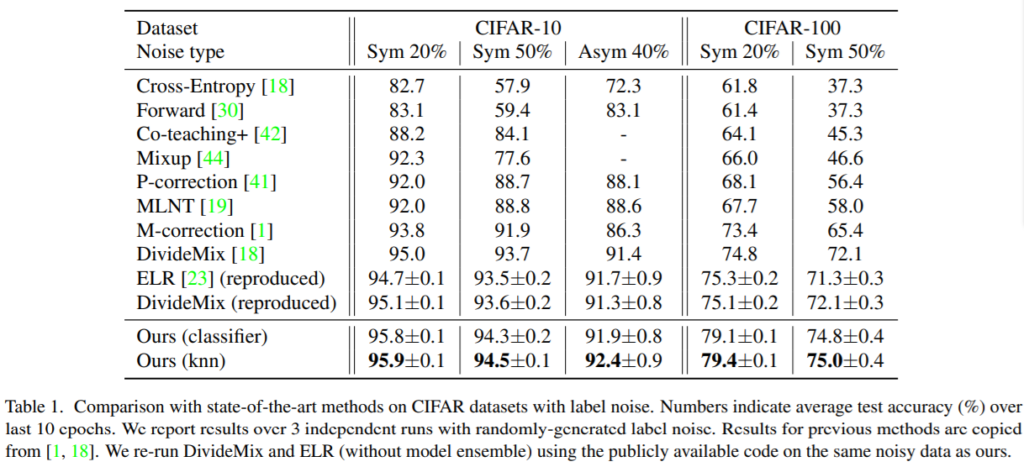
정성적 결과 제안하는 방식으로 학습을 진행하면 더욱 구별성 있는 feature embedding(method의 z space) 이 가능함을 t-SNE로 보였다
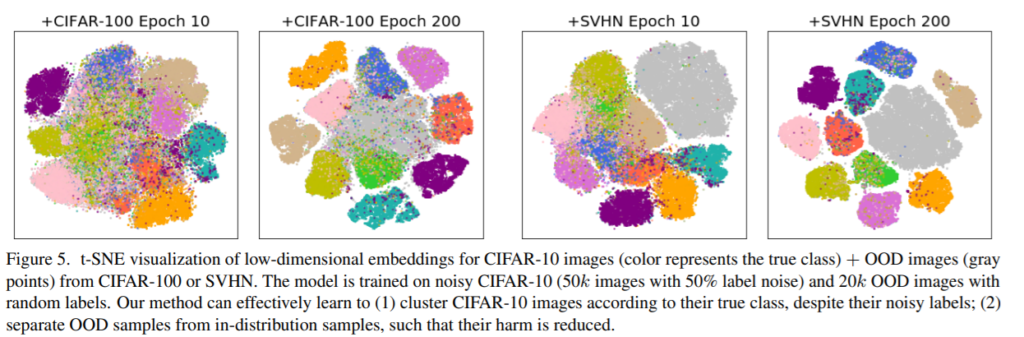
리뷰 잘 읽었습니다. ‘symmetric’ 이라는 말이 ‘대칭’이라는 뜻으로 알고 있는데, “이때 노이즈 생성을 위해 임의의 n% 데이터를 랜덤 선정하여 랜덤하게 라벨값을 바꾸는 Sym(Symmetric)방식” 에 symmetric 이라는 말이 어떤 의미로 들어가게 되었는지 궁금하여 질문 남깁니다.
노이즈 종류를 label noise / OoD / Input corruption로 세분화 하여 정의한거 같은데 혹시 각각의 노이즈 종류에 대한 실험 결과나 언급은 없나요?