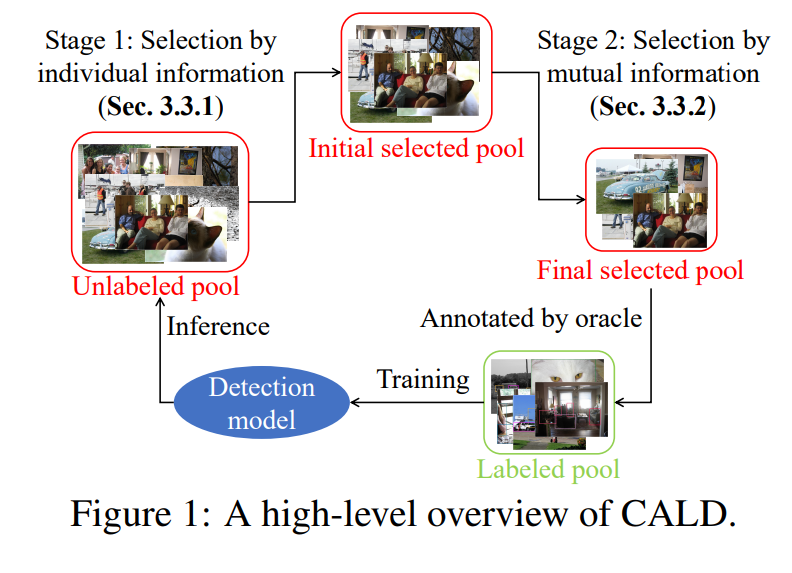
본 논문은 Active Learning에 관한 논문이다.
learning-based 알고리즘의 가장 큰 bottlenecks 중 하나는 labeled data의 부족이다. 이를 해결하기 위해 unlabeled, semi-supervised learning 기법들이 소개되었으나, 몇몇 방식은 실제 task 에 적용하기 어렵다. 이렇게 적은 labeled을 이용하는 방식 또한 연구되어야 하지만 실제 labeled dataset을 늘리는 방법에 대해서는 비교적 관심을 받지 못했다. 본 연구는 이러한 관점에서 annotation process를 효율적으로 진행할 수 있는 active learning 을 다룬다. (물론 active learning은 이 논문 이전부터 이미 존재하는 주제이다)
논문은 제안하는 방법론을 CALD (Consistency-based Active Learning method for object Detection ) 라 명명하는데 제목 그대로 object detection에 active learning을 적용할 수 있다. 기존의 active learning method는 image classification task에 적용이 많았다. 이러한 classification 기반의 active learning 연구를 object detection task에 직접 적용을 할 수 없었던 이유는 다음의 3가지와 같다.
- classification 문제와 object detection 문제의 관점 차이
classification은 class 예측에만 관심을 갖으면 되지만, object detection은 bounding box prediction에도 같은 중요도를 갖고 작동해야한다. - object detection 문제의 경우 informative object (정보량이 많은, 관심을 가져야하는, 선택되어야하는 물체)와 uninformative object가 같은 이미지에 존재할 수 있다.
- classification 문제에서 한 이미지에는 하나의 class가 존재하지만 object detection의 경우 하나의 이미지에 여러 object가 있어 multi class를 갖는다.
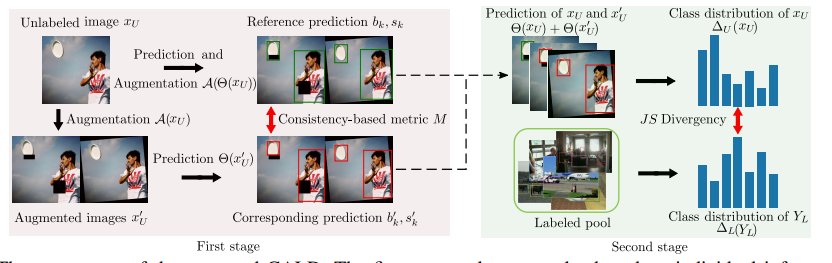
CALD의 진행과정은 2stage로 나뉜다. first stage는 individual information을 계산하여 initial selected pool을 생성하고 second stage는 mutual information(class 분포 정보)을 통하여 initial selected pool을 필터링하여 final selected pool을 생성한다.
1. Consistency-based Individual Information
stage 1에서 individual information을 계산하는 방법은 다음과 같다.
하나의 unlabeled image의 k 번째 prediction box와 그 점수를 bk,sk 그 이미지를 augmentation 후 k번째로 predict한 box를 bk’, 점수를 sk’라 하자.
이때 해당 k번째 예측의 consistency는 bk,sk와 bk’,sk’의 비교를 통해 구하며, 다음의 수식과 같다.
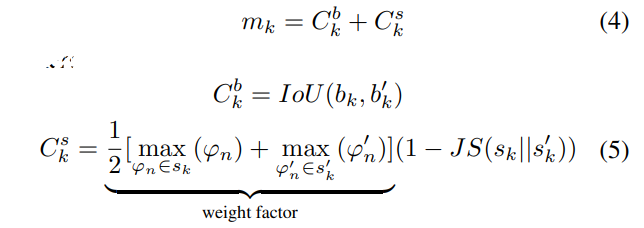
2. Mutual information
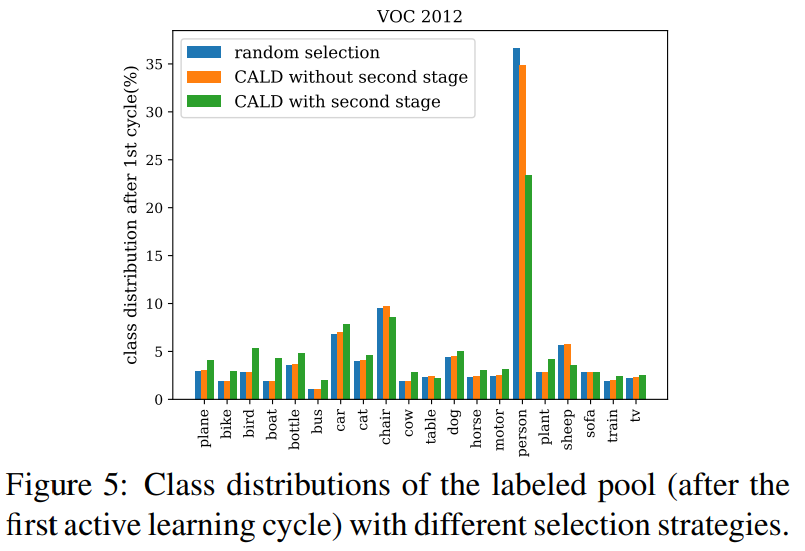
위를 통해 second stage를 통한 class 분포의 균형도 보정 효과를 확인할 수 있다.
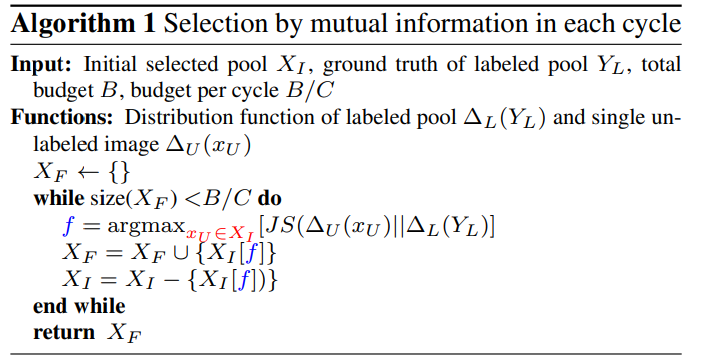
선택 방식은 다음과 같다.
요약
본 논문은 classification based active learning을 object detection에 적용하였으며, 두 task의 차이점을 정의하였다.
적용을 위해 stage 2에서 class unbalance 문제를 해결하였으며, stage 1에서 IOU를 도입해 task를 확장하였다