Spatial transformer Networks에 대해 리뷰하겠습니다. Pedestrian detection에서 ‘사람이 다양한 자세로 있을 수 있기 때문에 해당 개념을 적용하여 일반적 형태로 만들어 판단을 하면 사람을 더 잘 찾을 수 있지 않을 까?’라고 생각해 찾아봤던 논문입니다.
Abstract
Convolutional Neural Networks는 spatially invariant가 부족하다. 여기서 spatially invariant가 부족하다는 것은 transform, rotation, scale 변화 등 공간적 정보가 변했을 때 대응을 잘 못하는 것을 의미한다. 이 논문에서는 기존 CNN에 Spatial transformer 모듈을 추가하여 spatially invariace 하도록 한다.
Introduction
- 기존 CNN은 local max pooling을 이용해 공간적 변화에 대응했지만 pool size가 정해져 있고, 2×2와 같이 크기가 크기 않아 feature map크기가 클 경우 잘 대응하지 못했다.
- 이 논문에서 소개하는 Spatial Transformer module은 feature map 전체에 작용해 여러 공간적 변화에 대응할 수 있다.
그리고 영역을 찾아낸 다음 해당 영역의 이미지를 기준이 되는 형태로 transform한다. - 이 모듈은 CNN에 추가하기만 하면 back-propagation을 통해 end-to-end로 학습시킬 수 있다.
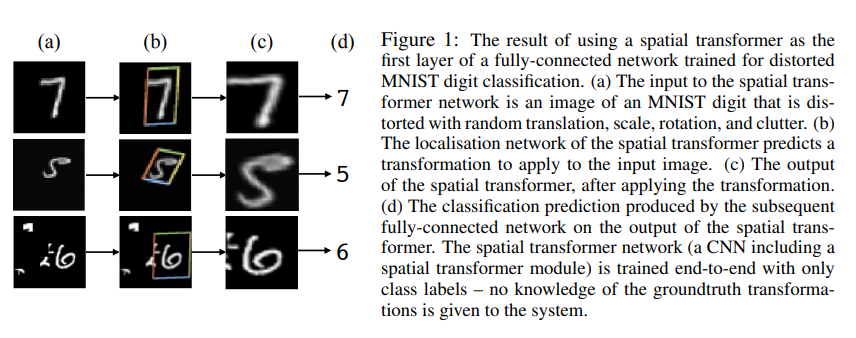
Spatial Transformers
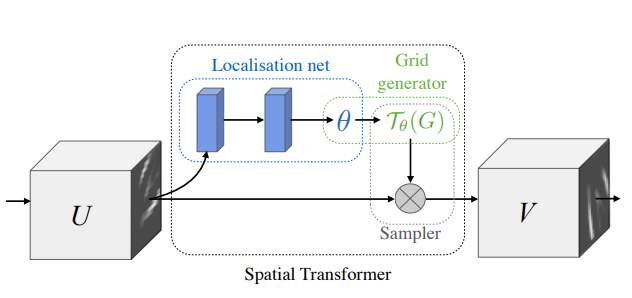
위 그림처럼 세 부분으로 나눌 수 있다.
Localisation network
- Localisation network를 통해 U에 적용할 transfrom parameter matrix θ를 추정한다. θ는 transformation종류에 따라 달라진다.
- network는 마지막 단에 regression layer가 있어야 한다는 것만 만족하면 네트워크는 convolutional layer든 fully-connected lyaer든 상관없다.
Parameterised Sampling Grid
grid generator
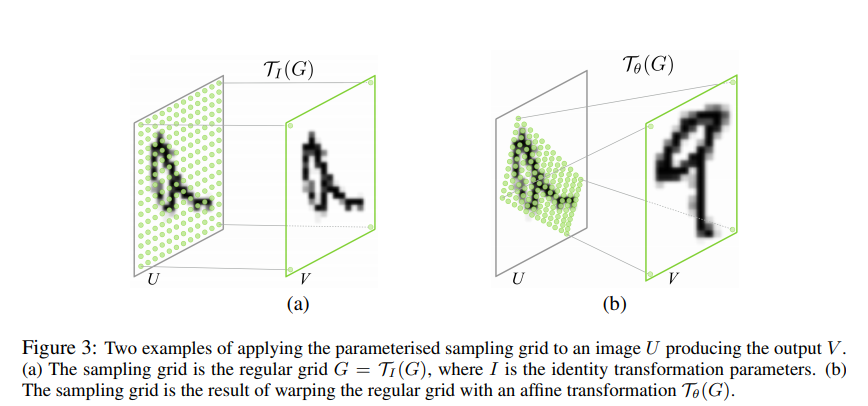
- θ 에 따라 U에서 sampling grid T_θ (G) 를 계산한다. 이때 V는 일반적 형태의 직사각형 그리드 위에 있고 V는 A_θ를 통해 U로 매핑된다.

scale, rotation, translation, skew, cropping을 표현하는 affine transfrom의 경우
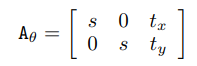
Differentiable Image Sampling
U에 T_ θ(G)를 적용해 V를 생성한다. 이때 T_ θ(G) 의 값은 U에서 어떤 점을 가져올 지를 나타내고 다음의 식으로 나타낼 수 있다.

이때 맵핑되는 픽셀의 위치가 정수가 아닌 경우가 많아 보간법을 이용해 값을 구해야 한다.
- Integer sampling
integer interpolation을 이용하여 인접한 정수의 값을 이용한 경우 다음 식을 얻을 수 있다.

- Bilinear sampling
bilinear interpolation (이중선형보간법)을 이용한 경우 다음의 식을 얻을 수 있다.

loss를 backpropagation을 통해 계산하려면 U와 G에 대해 미분 가능해야 하고 partial derivative는 다음과 같다.
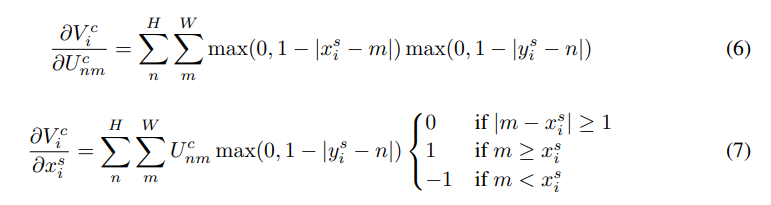
모든 구간에서 미분 가능하지 않은 경우 sub-gradients를 이용한다.
Spatial Transformer Networks
localisation network, grid generator, sampler로 구성된 spatial transformer 모듈을 CNN 구조에서 어느 지점에 몇 개를 집어넣어도 된다. 그리고 이 모듈은 학습 속도를 저하시키지 않는다. CNN의 입력 앞에 넣는 것이 일반적이다.
spatial transformer 모듈을 깊은 네트워크에 넣으면 추상적 표현을 transform할 수 있으며 localisation network는 더 많은 정보를 제공할 수 있다.
spatial transformer를 병렬로 사용하면 여러 객체나 attention에서 유용하다.
Experiments
Spatial Transformer Networks – YouTube 저자의 실험 관련 영상
- Distorted MNIST
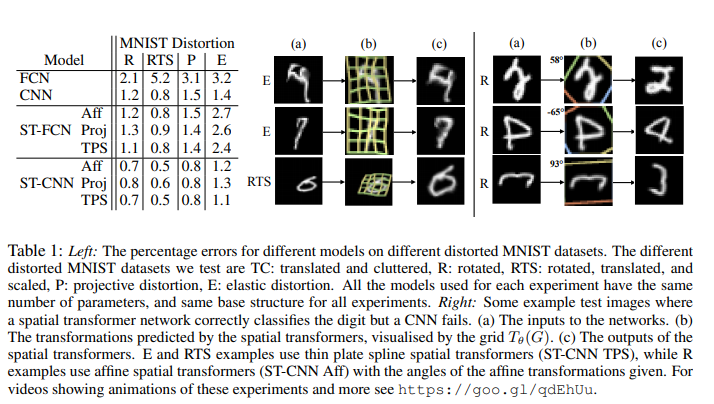
- distortion 종류 :
회전(rotation,R), 회전 및 크기 변환 (rotation,translation-scale, RTS), 투영(projective transformation, P), 탄성 왜곡(elastic warping, E) - FCN과 CNN, spatial transformer를 추가한 ST-FCNN과 ST-CNN 네트워크 비교함.
- bilinear sampling 이용
- transform 종류 :
affine transformation (Aff), projective transformation (Proj), and a 16-point thin plate spline transformation (TPS) - ST-CNN성능이 가장 좋았음.
- Street View House Numbers
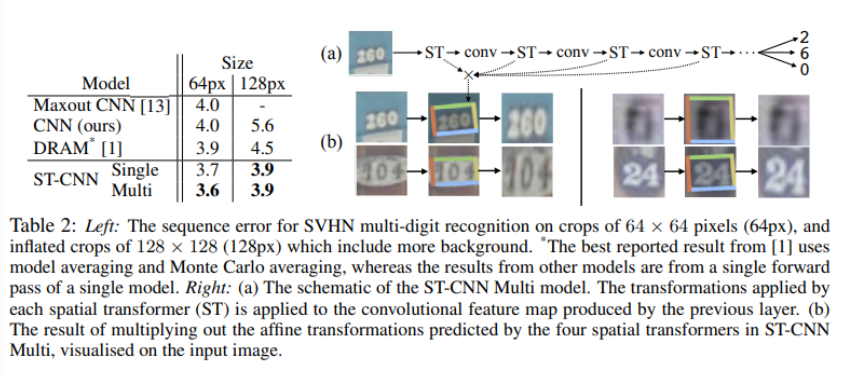
- Spatial Transformer를 1개만 추가한 single, (a)와같이 4개 추가한 multi
- affine transformation과 bilinear sampling사용
- ST-CNN의 multi 성능이 가장 좋았음. 이때, 속도 6% 저하
- Fine-Granied Calssification
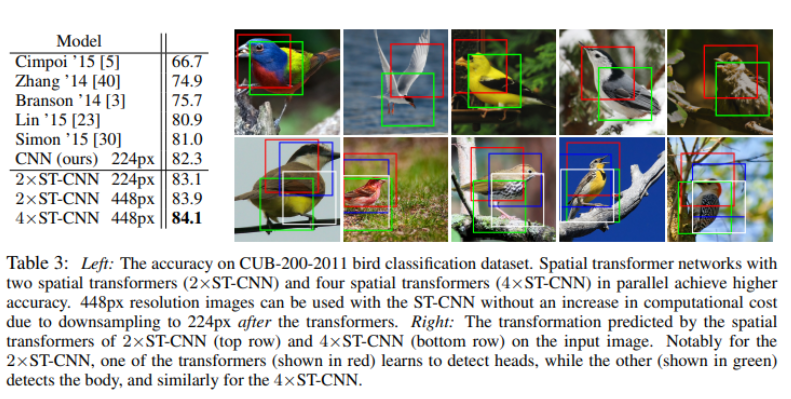
- ImageNet으로 pretrain되고 CUB로 fine-tuning된, batch normalisation이 있는 CNN모델을 베이스로 함.
- spatial transformer 2개와 4개를 병렬로 사용해 attention 하도록 학습ImageNet으로 pretrain되고 CUB로 fine-tuning된, batch normalisation이 있는 CNN모델을 베이스로 함.
- red는 머리, green은 몸통을 탐지하도록 학습됨.
conclusion
자체 내장 모듈인 spatial transformer는 네트워크 안에서 spatial transformation에 잘 대응할 수 있게 한다. 그리고 loss function을 변경하지 않고 end-to-end방식으로 학습할 수 있다. 해당 모듈을 추가하면 성능이 향상되는 것을 확인할 수 있다.
——————————————————————————-
논문에 다양한 실험과 자세한 설명이 많이 있습니다.
Pedestrian detection의 경우는 동일한 사람인지 인식하는 것이 중요한 것이 아니므로 spatial invariance가 중요한지 생각해봐야 할 것 같습니다. 그러나 해당 논문에서 공간적 변화를 인식해 일반적 형태로 나타내준다는 것은 pedestrian detection에서 활용할 만한 내용이라는 생각도 듭니다. 해당 논문에 대해 제가 잘못 이해한 부분이 있다면 피드백해주세요.